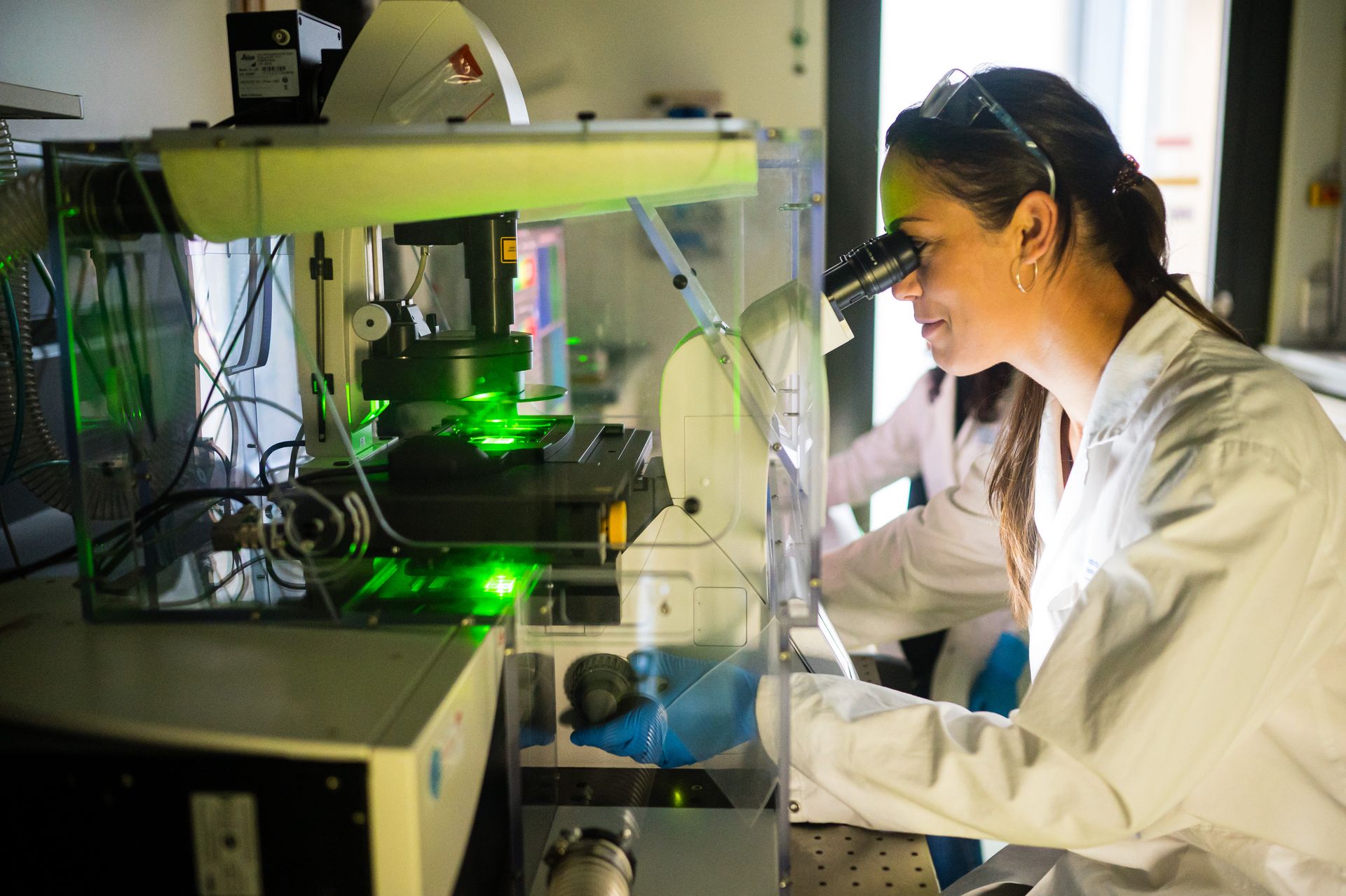
Clinical Bioinformatics
Prof Dr Andreas Keller
The Department of Clinical Bioinformatics group is concerned with analyzing molecular information using computer-based methods such as machine learning, artificial intelligence, or other algorithms. Its focus lies on spatially and temporally resolved processes to help understand how bacteria, as producers of natural products, interact with humans and can trigger or even protect against disease.
Our research and approach
The Department of Clinical Bioinformatics group focuses on computational methods for the analysis of nucleic acids, primarily RNAs. One focus of the research is on the regulatory mechanisms of non-coding RNAs and the impact of various influencing factors on the spatially and temporally resolved single-cell transcriptome. Based on the experience in modeling regulatory influences, the interaction of bacteria with humans is also investigated. Here, the exchange of information and material between commensal and pathogenic bacteria and their host is considered. The aim is to find new producers of natural products that can serve as a basis for the development of new active substances. The medical focus is to better understand and influence aging processes and neurodegenerative diseases of old age such as Alzheimer's and Parkinson's disease.
Team members

Prof Dr Andreas Keller
Group Leader

Anna Priester
Assistant

Sabine Lessel
Assistant

Annika Engel
PhD Student

Friederike Grandke
PhD Student

Pascal Hirsch
PhD Student

Victoria Wagner
PhD Student
Research projects
Spatial Single-Cell Transcriptomics
Together with the junior research group of Dr. Fabian Kern, affiliated with the clinical Bioinformatics Department, spatially and temporally resolved single-cell processes are investigated. Different tissue samples are analyzed and compared over their natural lifespan. In addition, the influence of pathogenic bacteria on organs is investigated in infection models and it is analyzed how drugs can change the cellular composition. Methods with a resolution of up to 500 nanometers will be used, allowing for the quantification of gene products even at the subcellular level. Artificial intelligence will be used to help computers learn to predict (sub)cellular changes that occur when drugs are administered to significantly improve in-silico ADME-Tox studies.
Clinical metagenomics
Bacteria can trigger a multitude of diseases in different parts of the body - or protect against them. In the IMAGINE project, various samples (eye, mouth, intestine, skin) are collected from patients (including those with chronic inflammatory bowel disease, diabetes, obesity, Parkinson's disease, lung tumors, and others), sequenced using shotgun sequencing, and analyzed on a computer using machine learning. From 3,000 patient samples so far, conclusions can be drawn about commensal and pathogenic bacteria and possible new natural products can be found. These are being investigated in the corresponding groups at HIPS to determine whether they are suitable as new drugs. In addition to the human microbiota, soil samples collected worldwide and animal samples from over 40 different species are also analyzed using the same methods to obtain a comprehensive picture of potential natural products.
Immune processes of neurodegenerative diseases
Impaired immune responses play an important role in neurodegenerative processes. Regardless of the trigger of the immune response, researchers in the Department of Clinical Bioinformatics want to understand how the immune response differs between patients and healthy individuals. To this end, T immune cells are collected from blood samples, stimulated, and then analyzed using single-cell sequencing. Machine learning methods will identify up to 30 different cell fractions and determine the change in profiles over time between patients and controls for each cell fraction. This allows for deciphering how immune cell types contribute to corresponding pathological processes. This in turn represents a starting point for new therapies.
Regulatory effects of non-coding RNAs
Non-coding RNAs interact with genes that contain the blueprint for proteins. Even small changes can result in serious physiological consequences when the existing regulatory balance is disturbed. By combining high-throughput reporter assays and systems biology modeling, it can understand how non-coding RNAs control gene expression networks in an organized fashion. Based on the models, we are working to restore the balance that originally existed. In addition, we are exploring how non-coding RNAs of bacterial origin can specifically regulate genes in humans. Such RNAs are also candidates for new drugs.