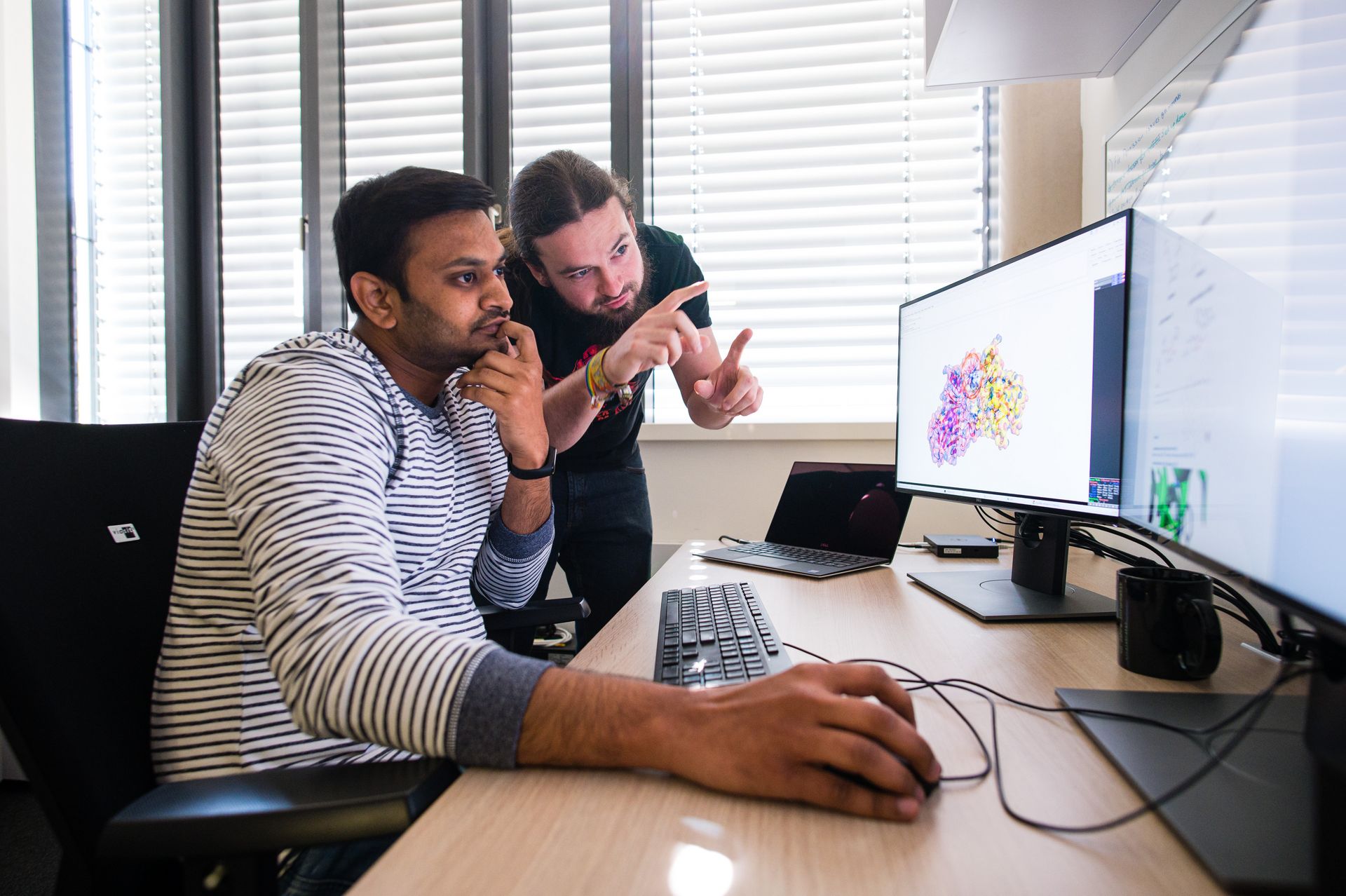
Spatiotemporal single-cell Bioinformatics
Dr Fabian Kern
Infections and therapies using anti-infectives involve diverse spatially and temporally dependent biochemical processes with numerous cell type related mechanisms yet to discover. We develop progressive bioinformatics-driven approaches, as for example domain-specific neural networks based on spatiotemporal RNA profiles, for the rapid and comprehensive analysis of healthy or diseased cell functions. Advanced computational models will allow us to gain a deeper mechanistic understanding of cellular signaling cascades and pathways underlying infectious diseases. We thus aim to promote from the computational side the translational process for compounds developed at HIPS toward successful clinical applications and novel therapies.
Our research and approach
High-throughput sequencing and ultra-efficient bioinformatics software drive most of current academic and industrial branches in biomedical research. Essential factors of bacterial or viral pathogenicity, for instance virulence or drug resistance mechanisms are governed by complex gene-regulatory pathways as well as the type of host cell and pathogen, which can be elucidated by sequencing. The underlying molecular processes leading to observed clinical phenotypes can then be deciphered using AI- and bioinformatics-driven methods. On-going research on antibiotics, anti-infectives and resistance mechanisms increasingly utilizes spatially and temporally resolved single-cell readouts, revealing to us promising new intra- or extracellular targets of interest.
The research group led by Dr. Fabian Kern aims to identify such targets using deep learning and big data analytics techniques, amongst others utilizing data from lab infection models and human clinical samples, performed in close collaboration with partners from the HIPS, the Department of Medicine as well as the Department of Natural Sciences at Saarland University. In detail, we are interested in gaining a better understanding of host infection responses at important biological interfaces such as the gut or blood-brain barrier. Our long-term goal is to identify those cellular changes over space and time which are initially caused by infections, by using interpretable machine-learning models and graph-based algorithms. To this end, we contextualize molecular data sets with demographic, clinical and individual features as well as drug compound characteristics. In this way, we eventually try to enable a better understanding of complex human phenotypes like accelerated aging or chronic diseases of the elderly, that may in parts be related to preceding phases of severe infection.
Team members

Dr Fabian Kern
Group Leader

Matthias Flotho
PhD Student
Research projects
Machine learning from state-of-the-art spatial and single-cell transcriptomics technologies
The rapid development of (RNA-)sequencing assays at unprecedented throughput and resolution enables us to capture detailed molecular profiles for millions of single cells from human tissue or body fluids, and even for single disease-causing germs. Recent experimental approaches combining methods to individually tag RNA molecules from single cells at specific tissue locations for subsequent high-throughput sequencing open a myriad of potential viewpoints to study the spatial patterns of infectious disease (Spatial Transcriptomics). For a deeper analysis of sequencing data and to discover functional relationships advanced integrative bioinformatics tools are required. Moreover, several known but also yet hidden technical factors in such experiments play an important role during data generation and influence our machine learning-driven interpretation of outcomes. Thus, we make use of different state-of-the-art technologies (e.g. Visium, STOmics) in close collaboration with leading companies in the field to test our computational approaches under varying experimental setups. Primarily we are looking for interesting and challenging applications around infection, neurodegeneration and antibiotics research as to further cartography cellular and molecular features as a basis for new drug target candidates.
Developing graph-based methods to predict cellular neighborhoods and signaling pathways
To facilitate the generation of AI-ready and machine learning compatible data sets, we make use of the available domain knowledge at and around HIPS to add further required levels of abstraction to our experimental data. To this end, we are searching for new computational approaches, e.g. hashing, to quickly distinguish pathogenic from healthy cell profiles in big data sets and to reveal unique disease signatures. Modelling cells or communities of such as nodes in global graphs is a promising approach that recently received more attention in the field. Edges between the cell nodes are determined, for example by ligand-receptor pathways or other biochemical processes typically governed by gene expression. Our methods aim to discover also reproducible and testable gene signatures (so-called panels) deciphering those parts of the transcriptome specifically afflicted by disease-causing germs. In principle, such panels can then also be used to judge the mechanism of action of an existing or new drug compound, which ideally at least to some extent reverses degenerated gene regulation programs back into a healthy and homeostatic state.
Identification of actionable disease markers at biological interfaces to derive new pharmaceutical compounds
Biological barriers are an essential mean of the human body to establish certain physiological conditions and play an important role for our immune system. First and foremost, they keep individual tissue compartments separated and different zonation areas sterile, i.e. free from circulating microbes. Numerous examples of human diseases exist where impaired bio-interfaces are one of the major underlying pathogenic entry points. At the same time, an on-going challenge in drug development is to transfer small or natural compounds beyond these barriers at a specified time and concentration. Thus, we are interested to uncover gene expression programs of the often very specialized cells, like epi- or endothelial cells, as well as peripheral immune cells residing at tissue gateway interfaces and how we can use them for targeted drug delivery. Furthermore, we see strong potential in a class of compounds that is able to molecularly repair dysregulated barrier cells, eventually supporting human therapy.
Implementation of publicly available and free-to-use scientific databases and web servers
Bioinformatics routinely involves processing enormous amount of data of various flavors. Overall, there is a strong trend for exponentially increasing data amounts and assay complexity. Thus, among our scientific community we are in urgent need of open, flexible, and highly efficient formats for data storage and exchange. Our group follows the FAIR principle and relies on our strong experience on developing broadly used and peer-reviewed online resources like databases and web servers. We continuously share new software and experimental data sets with the scientific community and place value on providing sufficiently preprocessed and comprehensive data collections in order to be AI-ready. Finally, we commit ourselves to enable transparency and reproducibility in the field and thus also invest research into how we can improve computational tools in those nowadays essential aspects. To reach this goal we run joint collaborations with our Helmholtz colleagues from the CISPA Helmholtz Center for Information Security.
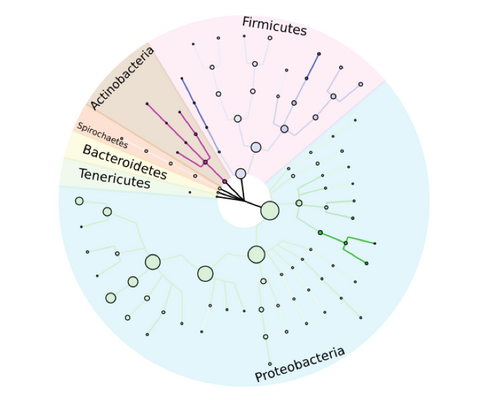
With the aid of large plasmid sequence databases, a detailed taxonomic tree of bacterial strains can be reconstructed. Figure adapted from our publication: Georges P Schmartz, Anna Hartung, Pascal Hirsch, Fabian Kern, Tobias Fehlmann, Rolf Müller, Andreas Keller, PLSDB: advancing a comprehensive database of bacterial plasmids, Nucleic Acids Research, Volume 50, Issue D1, 7 January 2022, Pages D273–D278, https://doi.org/10.1093/nar/gkab1111. © Oxford University Press